The Distinction Between Generative AI and AI
Artificial intelligence (AI) has become a prominent force in our lives, transforming the way we interact with technology and shaping various industries. With the constant advancements in AI, there has been a growing interest in understanding the distinction between generative AI and traditional AI. Although both are subsets of AI, they differ in their approach and capabilities. In this article, we will unravel the differences between generative AI and AI and explore their applications and challenges.
Thesis statement: Understanding the distinction between generative AI and AI is crucial in comprehending the advancements in AI and their potential impact on various industries.
Before diving into the distinction between generative AI and AI, it is essential to understand two key concepts: machine learning and natural language processing (NLP). Machine learning is a subset of AI that allows systems to learn and improve from experience without being explicitly programmed.
On the other hand, NLP is an AI-based approach that allows machines to understand, interpretand generate human language. Both these concepts serve as the foundations for generative AI and AI.
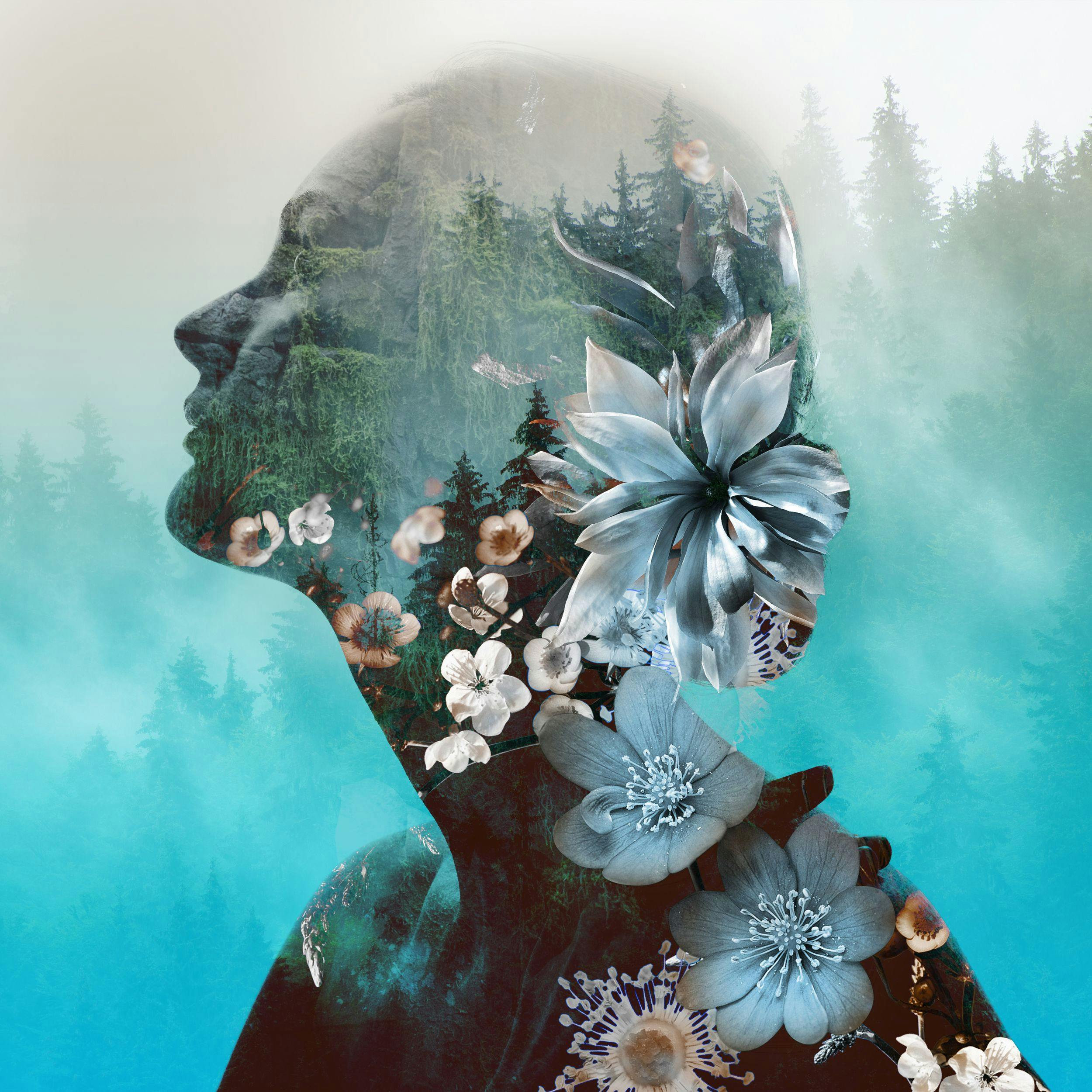
Artwork by Sean M. Smith
What is the difference between generative AI and traditional AI?
The traditional approach to AI involves designing algorithms to perform specific tasks by extracting patterns from data. These algorithms are generally based on statistical models and rules. In contrast, generative AI focuses on creating new content or data rather than just analyzing existing data. It seeks to understand the underlying structure of data and use that knowledge to create new data that resembles the original. In simpler terms, traditional AI solves problems, whereas generative AI creates new solutions.
How does generative AI work and what are its applications?
Generative AI uses deep learning algorithms to process vast amounts of data, detect patterns and create new outputs. These algorithms are trained using a large dataset and can then generate new samples that resemble the original.
This capability has led to various applications in industries such as media and entertainment, fashion and gaming. For instance, in the media and entertainment industry, generative AI is used to create realistic video game characters, animationand special effects. In fashion, it aids in designing custom clothing based on customer's preferences and body measurements. Moreover, in gaming, it creates unique and dynamic environments and characters, making the gaming experience more immersive.
Can generative AI algorithms be used for tasks where traditional AI methods have failed?
One of the significant advantages of generative AI is its ability to learn and adapt to new situations by continuously generating new outputs. This makes it ideal for tasks where traditional AI methods have failed. For example, traditional AI may struggle to understand creative or abstract concepts as it relies on data and rules. However, generative AI can observe data, learn the patterns and create new outputs even for abstract or creative ideas. This capability makes it useful for industries like art and music, where abstract concepts and creativity are vital.
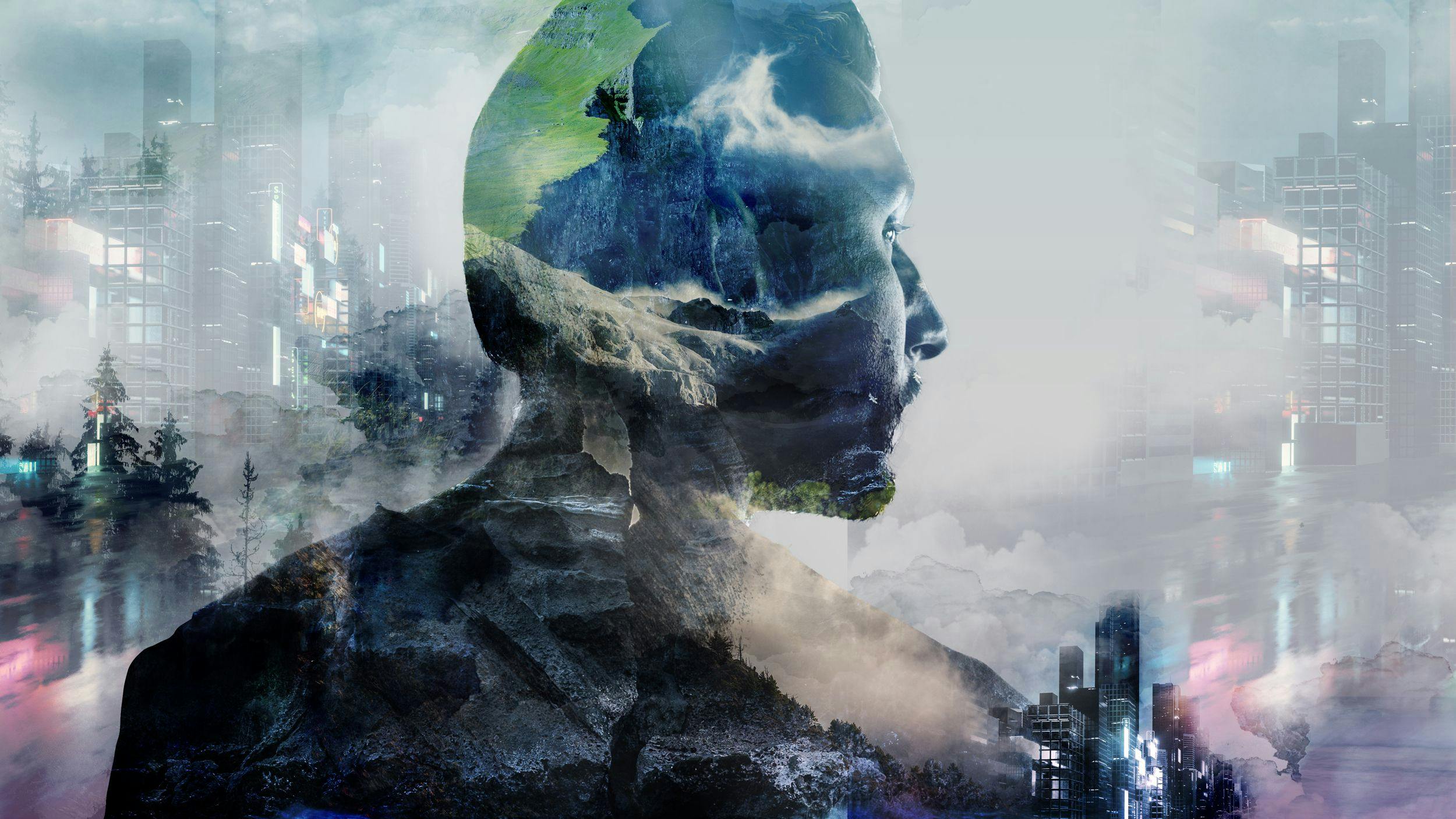
Artwork by Sean M. Smith
What are the main challenges in developing generative AI and how are they being addressed?
As with any emerging technology, there are challenges in developing generative AI. The primary challenge is the "black box" problem, where it is challenging to interpret and explain how the algorithm arrived at a particular output. This raises concerns about transparency, especially in critical applications like healthcare and finance. To address this challenge, researchers are working on developing techniques to explain and visualize the decision-making process of generative AI algorithms.
Another challenge is the data dependency of generative AI algorithms. These algorithms require significant amounts of data to learn from, which can be a challenge in some industries. To overcome this, researchers are exploring ways to reduce the data dependency of generative AI and develop algorithms that can learn with fewer data.
Laetro.com is an online platform that enables businesses to create custom AI applications by leveraging the power of generative AI. It offers a user-friendly interface and a variety of pre-built templates, making it easier for businesses to implement generative AI without extensive knowledge of AI or coding.
In conclusion, understanding the distinction between generative AI and AI is essential in comprehending the advancements in AI and their potential impact on various industries. Generative AI goes beyond traditional AI by creating new content and this has led to its various applications in industries like media, fashion and gaming.
However, there are still challenges in developing generative AI, such as the "black box" problem and data dependency, which are being addressed by researchers. With platforms like Laetro.com, integrating generative AI into businesses has become easier, paving the way for its widespread adoption.
To summarize, here are the key points discussed in this article:
- Generative AI differs from traditional AI by creating new content rather than just analyzing existing data.
- It uses deep learning algorithms to generate new outputs.
- Generative AI finds applications in industries like media, fashion and gaming.
- It can be used to tackle tasks where traditional AI methods have failed.
- Challenges in developing generative AI include the "black box" problem and data dependency.
- Laetro.com is an online platform that makes it easier for businesses to implement generative AI applications.